AutoEPC
Explainable AI for fast predictions of Energy Performance Certificate (EPC) ratings.
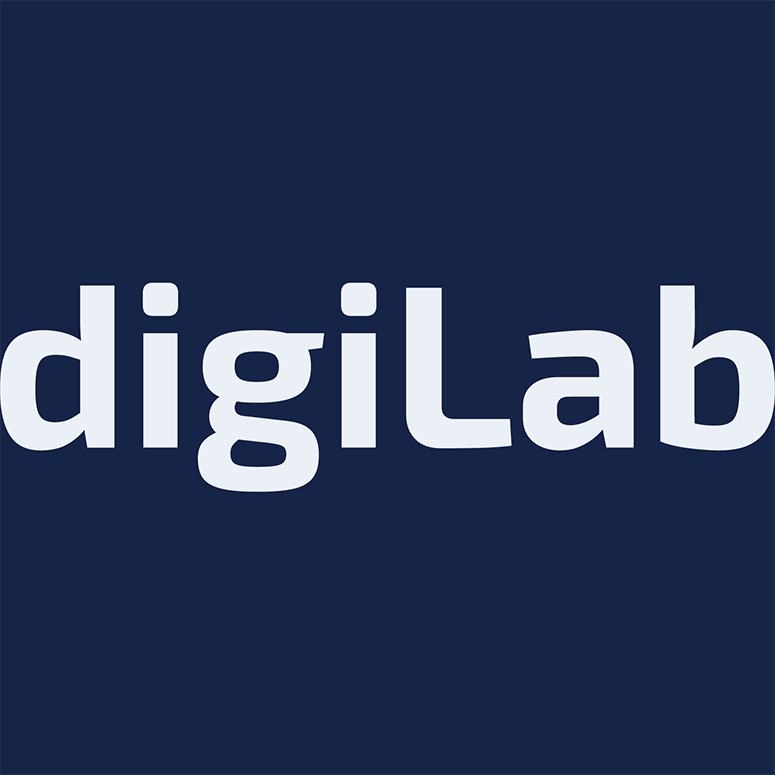
Project
AutoEPC
Lead Organisation
digiLab
URL
Location
Exeter
Funding
£174,408
About the project
EPC ratings are expensive to commission, difficult to complete and adhere to moving regulatory goal posts, meaning legacy records an a poor metric of Energy Performance. Our goal is to use machine learning to quantifiable rectify this:
- We are building a tool to measure EPC scores cheaply and with statistical confidence.
- We seek to reduce the complexity of input information and to provide a measure of audit behind the reduced-order calculations.
- We will provide a mechanism to rapidly reevaluate and rectify important legacy data on a regional basis.
At digiLab we are building an enterprise product to execute AI workflows: this is called twinLab. The workflow that we are cultivating in this project is targeted to solve extremely expensive EPC-related problems for rapid decision intelligence.
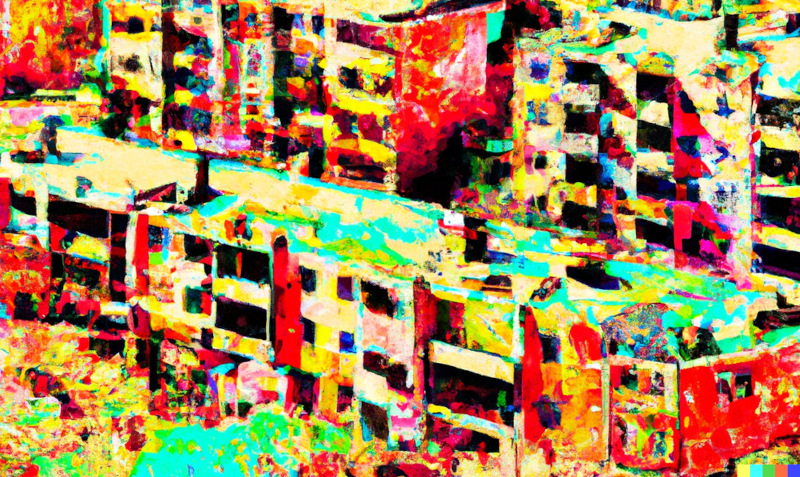
What has the project successfully delivered?
- An explainable machine learning algorithm for the prediction of EPC scores based on easily computed features.
- Web-interface in development for non-technical users of the model.
- An API service to allow technical customers to integrate the service into their workflows.
What has the project achieved?
Route to a tangible software as a service (SaaS) product which improves efficiency using machine learning.
Key lessons learned
EPC ratings as an objective measure of energy performance are a poor metric. Amending this standard requires significant research and government intervention. Our present tool lays the baseline for what can be achieved within the parameters of the existing EPC formalism. It also supports the use of machine learning in future regulatory frameworks for energy performance as a means to build accurate and easy-to-use tools.
Next steps
Market analysis and client engagement to cement our tool in the workflows of organisations.